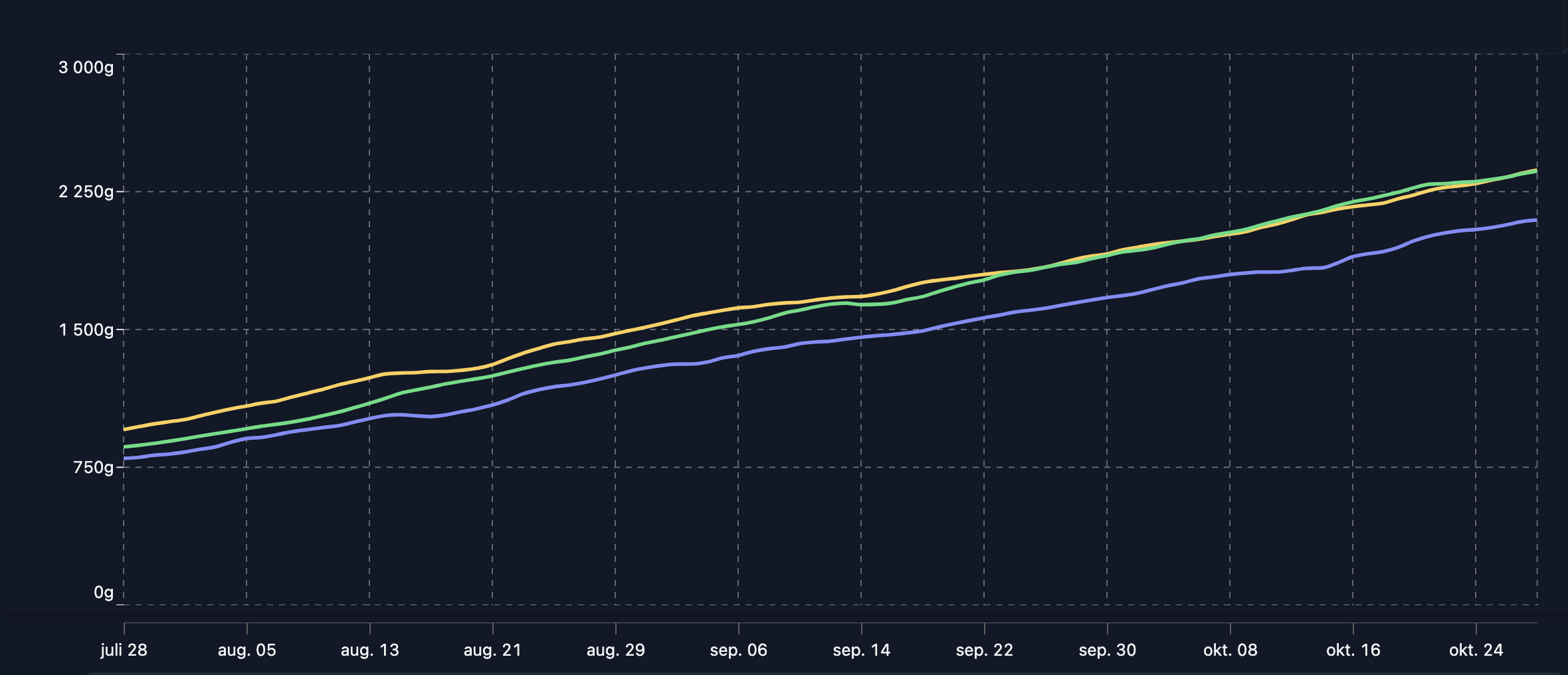
Better decisions with accurate data on biomass, weight, and growth
With accurate calculations of weight and biomass, the insights from Aquabyte WEIGHT serve as a decision-making foundation when critical choices must be made during production. The module provides updated data daily, making it easy to track developments in biomass, weight, weight distribution, and K-factor. Weight forecasts up to two weeks ahead, based on historical and environmental data, enable Aquabyte WEIGHT to generate detailed and accurate harvest reports.
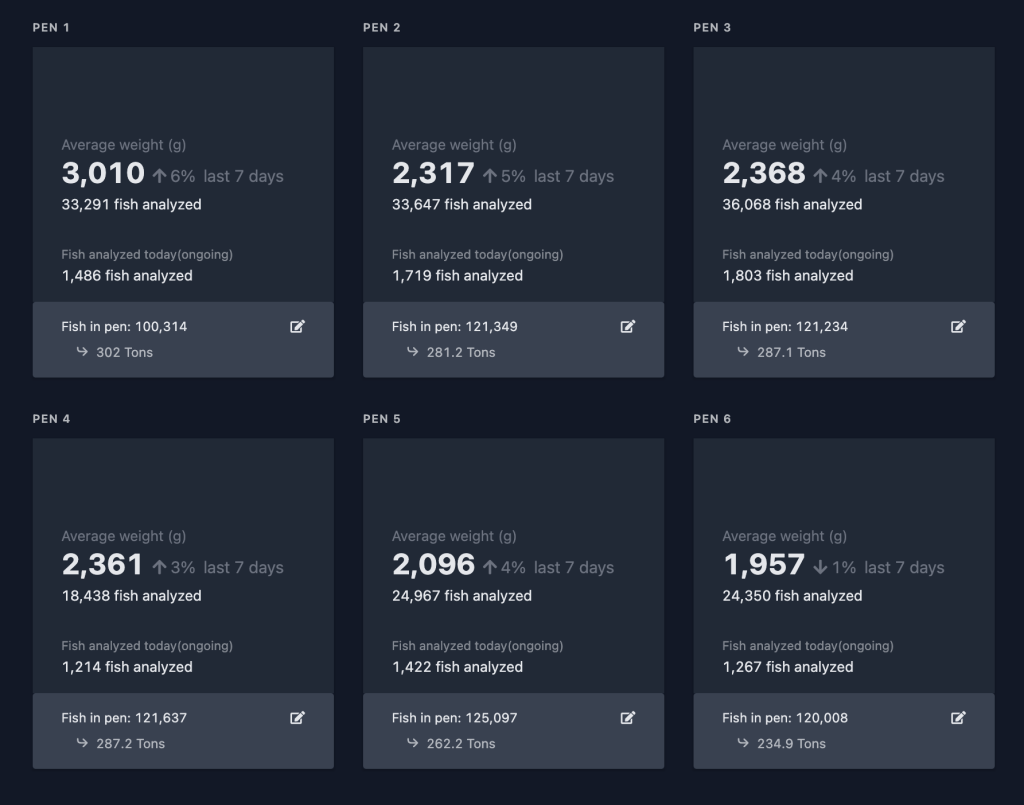
Benefits of using Aquabyte WEIGHT
- Automated weight calculations reduce stress and injury to fish
- Key decisions can be made based on insights rather than assumptions
- Feeding and feeding strategy can be optimized according to actual weight and growth rate
- Accurate harvest reports generated up to two weeks before harvesting
- Better predictability in future weight development and profitability
Weight calculation based on images and machine learning
The weight calculations performed by Aquabyte WEIGHT are based on thousands of images taken daily by the Aquabyte camera within the pen. These images are analyzed using artificial intelligence and machine learning, providing precise calculations of key factors such as weight, growth, and biomass. The data retrieved from the pens is presented in the Aquabyte User Portal.
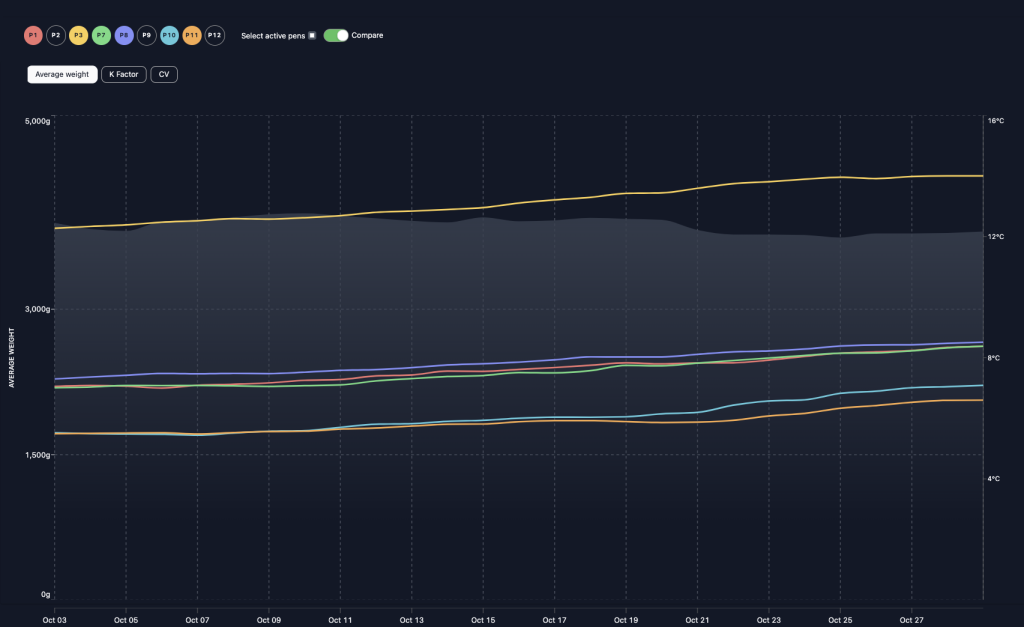
Overview with trendlines and graphs
Data on average weight, growth, fish population and total biomass are presented to provide an easy overview of each individual pen and allow status comparisons across different pens and sites. Detailed information on the development of average weight, K-factor, and coefficient of variation (CV) is displayed in charts with data going back up to 12 months. Weight distribution in actual weight is also shown as bar charts, either for a single pen or multiple pens.
All weight calculations, graphs, and charts are updated daily in the Aquabyte User Portal. Together, they provide insights into both current status and trends over time, serving as a valuable decision support tool when critical choices need to be made during production.
Optimize feeding
The daily updates provided by Aquabyte WEIGHT are an excellent tool for optimizing feeding. Accurate data on biomass, weight distribution, and growth development provide insights into the fish’s actual status, allowing for early detection and correction of over- or underfeeding. Optimized feeding based on accurate weight data saves the company significant expenses and creates real value for the business in both the short and long term.
Accurate harvest reports
With two-week growth forecasts, Aquabyte WEIGHT generates highly accurate harvest reports, creating predictability for both the site and the harvesting plant receiving the fish. The harvest reports are based on data from the production period, the last day of feeding, water temperature, and other factors that impact fish growth in the upcoming period. The reports calculate round weight, HOG weight, and estimate weight distribution within the pen.
When Aquabyte WEIGHT is combined with Aquabyte WISE Welfare, the harvest reports also provide information on the estimated percentage of superior-grade fish per pen.
Custom downgrading criteria
Aquabyte WEIGHT allows for defining custom downgrading criteria for harvest reports. Criteria can be set according to company standards or tailored to the specific requirements of the harvesting plant receiving the fish. Downgrading criteria are saved in Aquabyte WEIGHT and can be selected for each individual harvest report to be created.